Resting state EEG functional connectivity analysis in children with benign epilepsy
Benign childhood epilepsy (BCE) is one of the most common idiopathic epilepsy with prevalence rates between 10 to 20% in children with epilepsy. Unlike other types of epilepsy, benign epilepsy is known to be unaffected by brain structural abnormalities. Despite the focal nature of benign epilepsy, there is growing evidence of disturbancse in memory, language, attention, auditory and cognitive processes in BCE patients. This implies that BCE is associated with abnormal network organization of brain areas. In two studies, we investigated differences in resting-state EEG between BCE patients and healthy controls under the eyes-closed condition. We also studied the impact of interictal epileptic discharges (IEDs) on functional connectivity patterns in BCE patients. To this end, we used graph theory metrics to characterize functional connectivity in brain networks.
Study I
We investigated changes in the brain functional connectivity in BCE patients with compared to healthy controls in various frequency bands using high-density resting EEG data under the eyes-closed condition. We also explored the impact of IEDs on the funtional organization of brain networks in BCE patients. High-resolution EEG recorded from each patient and healthy subject were first filtered between 0.5 to 30 Hz to exclude high-frequency noise. Artifact-free portions of the EEG data were then partitioned into two-second non-overlapping segments. EEG segments were randomly selected for each of control subjects (CON) and epileptic patients under two conditions: EEG segments with interictal spikes (With Spike Condition - WSC) and spike-free segments (No Spike Condition - NSC).
Pairwise correlations between all EEG channels were computed with Phase Locking Value (PLV). To calculate the PLV, the data was first filtered into frequency bands (δ (0.5–3.5 Hz), θ (4–8 Hz), α (8.5–13 Hz), β1 (13.5–20 Hz) and β2 (20.5–30 Hz). The end-result was connectivity matrices, including 63 vertices (electrodes) connected by edges between different pairs of channels. Functional connectivity matrices were converted to binary graphs by applying an optimal threshold determined under two conditions, (i) the mean degree must not be less than 2ln(N), and (ii) at least 95% of nodes must be connected to one or more nodes. To investigate the global topology of large-scale brain functional networks in patients and controls, network metrics such as degree (K), clustering coefficient (C) and path length (L) were then estimated from functional matrices as a function of network density. Nonparametric permutation testing was used for all graph parameters with correction for multiple comparisons, including post hoc tests with a p-value ≤ 0.05. A total of 1,000 permutations were used to determine the significance level for each test.
Our results showed that brain functional connectivity patterns in BCE patients were disrupted in terms of synchronization and degree of connectivity in both the IED zone and in frontal and temporal areas. Deviations from small-world features and network parameters were also observed in various frequency bands in BCE patients regardless of the presence of spikes in EEG segments. In WSC compared to CON, degree significantly increased in the right centrotemporal region and decreased in the occipital region in almost all frequency bands. Right frontocentral areas exhibited lower degrees in mid-range frequencies (θ and α). On the contralateral side, however, the degree significantly increased in the left frontal and frontotemporal regions in the θ band. In the absence of IED (NSC vs. CON), K increased in the left frontal region in the θ band and in right centrotemporal areas in high frequencies (β1 and β2). In the mid- and high-range frequencies, patients exhibited relatively lower degree values in occipital areas.
Compared to NSC, WSC was characterized with significant increases of K in the right centrotemporal regions in the δ and α band, and in the right parietotemporal regions in the β1 band. The degree decreased significantly in occipital areas in all frequencies except in the θ band, in the right frontocentral region close to the spike generation zone in mid-range frequencies (θ and α), and in the left posterior region in the β1 band (Fig. 1). In the δ band, patients under the WSC condition exhibited higher C and longer L compared to controls, implying that, at very low frequencies, BCE brain networks exhibit more functionally ordered organization in the presence of IED. Interestingly, a switch in the brain network functional organization in the θ and α bands was observed when comparing WSC and NSC conditions. BCE patients’ brain networks tended to change from a more randomly organized network (low C, short L) in the α band to a functionally ordered network (high C, long L) in the θ and β bands due to IED. The global increase in C in the presence of IED (excluding the α band) reflects orderly connections of IED brain networks. In the absence of IED, C was lower in all frequency bands and L was lower in the θ and β bands in BCE patients compared to controls, indicating that, in the absence of IED, the BCE brain network is less ordered in almost frequency bands except in the α band. Overall, we found frequency-dependent small-world features and network parameters in BCE patients regardless of the presence of IED in EEG segments (Adebimpe et al., 2015).
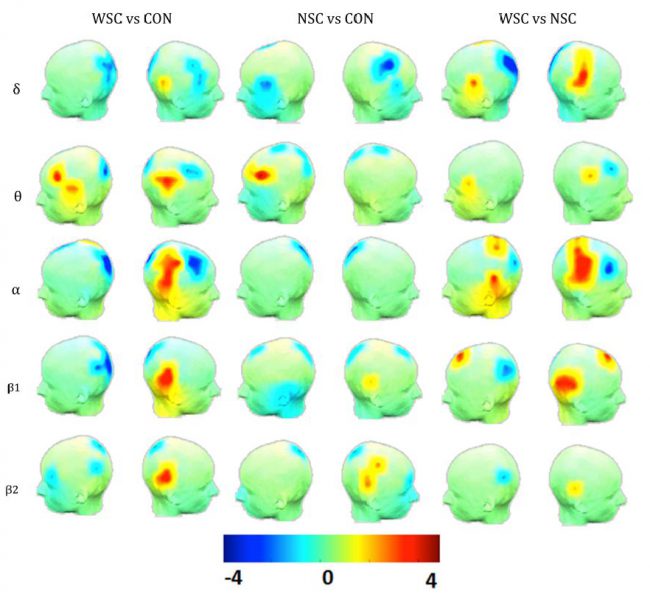
Figure 1. T maps of degree between controls (CON) and the epileptic group (WSC and NSC). The significant increase (indicated by red) and decrease (indicated by blue) in degree have been represented, respectively, by positive and negative t values resulted from statistical comparisons between (WSC and CON), (NSC and CON), and (WSC and NSC).
A potential limitation of this study was the use of scalp EEG for the functional connectivity analysis. The functional connectivity analysis in sensor space might provide inaccurate information on the funtional organization of cortical regions mainly because EEG electrodes detect spatially averaged overlapping signals from several brain sources.
Study II
In the second study, we investigated changes in brain functional connectivity in BCE patients compared to healthy controls in various frequency bands under the eyes-closed resting condition. Functional patterns of resting-state EEG source connectivity in patients were determined by lagged phase synchronization, a nonlinear connectivity measure implemented in the eLORETA software (http://www.uzh.ch/keyinst/eLORETA/). Graph theory was also used to characterize functional connectivity by estimating network centrality, functional segregation and integration. We used the same EEG database that was used in our previous study. The artifact-free portions of EEG data were partitioned into two-second quasi-stationary segments required for spectral analysis with a frequency resolution of 0.5 Hz. Five EEG segments were randomly selected for each patient (PAT) and healthy control (CON). The EEG segments selected for patients included no centrotemporal spikes.
EEG source connectivity analysis was then performed using eLORETA by restricting the source space within the gray matter including 6239 voxels with a 5-mm spatial resolution. The Montreal Neurologic Institute average MRI brain (MNI152) with anatomical labels corresponding to Brodmann areas was used as the realistic head model to compute the lead field. The 84 commonly used Brodmann areas were chosen as regions of interests (ROIs) for connectivity analysis between the centroids of the ROIs. To analyze functional connectivity we computed lagged phase synchronization (LPS) (Pascual-Marqui, 2007b) between ROIs. For each subject, FC matrices were computed in four frequency bands, δ (0.5 – 3.5 Hz), θ (4 -8 Hz), α (8.5 – 13 Hz) and β (14-30 Hz). This connectivity measure is resistant to artifacts of volume conduction by excluding the instantaneous zero-lag contribution (Canuet et al., 2011; Vinck et al., 2011).
For each subject and each frequency band, an average FC matrix was obtained over five EEG segments selected for the subject and was used to compute graph metrics. From each FC matrix, we extracted three graph measures to investigate functional integration and segregation between brain networks in patients compared to controls: network degree (K), global efficiency (E) and clustering coefficient (C). Each graph measure was calculated by the average over the whole nodes for a wide range of thresholds from 0.2 to 0.7 with an increment of 0.05 to investigate differences between the two groups. Group differences in functional connectivity and global network metrics were statistically evaluated using non-parametric permutation t-tests with p<0.05 (Bonferroni corrected for multiple comparisons). We further used post hoc tests to explore the directionality of effects over conditions with p < 0.05. A total of 1,000 permutations were used to determine the significance level for each test (Maris and Oostenveld, 2007). The results were then projected onto a 3D surface using BrainNet (Xia et al., 2013).
Our results showed that the functionality of brain networks in BCE patients was altered, particularly in the α and β bands. Compared to healthy subjects, BCE patients were characterized by widespread higher and lower phase synchronization values (Fig. 2) in α and β bands, respectively. In θ and α bands, significantly lower global clustering coefficient and higher network degree and efficiency were observed in patients. In contrast, compared to controls, patients displayed significant lower global metrics in β band. In δ band, no significant differences in global graph metrics were observed between patients and controls.
We also found that the interictal state in BCE patients is less ordered, displaying lower segregation (lower global clustering coefficient) and higher integration (higher global efficiency and network degree) in θ and α bands (Fig. 3). In α band, the lower clustering coefficient and the higher network degree might be due to the loss of local connectivity between neighbour nodes in comparison to increased long distance connections in BCE patients. In β band, we observed less long- and short-distance functional connections in BCE patients, who exhibited reduced clustering coefficients (representing functional segregation) and lower global efficiency and network degree (representing functional integration) compared to healthy controls.
Our functional connectivity analysis at Brodmann areas revealed higher network degree and clustering coefficient in epileptogenic areas, including centrotemporal, premotor, and somatosensory regions in θ and α bands in patients. We also found higher degrees in the posterior cingulate cortex in patients in the θ and α bands. Our results also confirmed higher phase synchronization values in regions with increased local segregation neural processing (high clustering coefficient), especially in the primary motor, postcentral and posterior cingulate regions (Adebimpe et al., 2016).
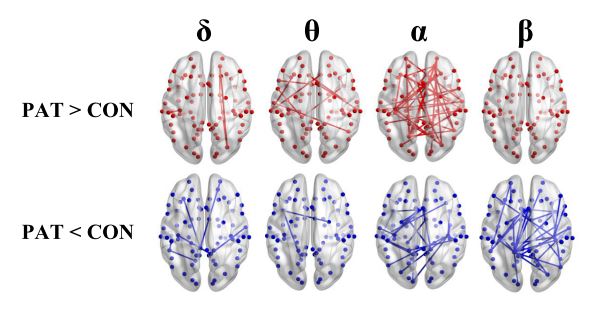
Figure 2. Results of the functional resting-state source connectivity analysis with the seeds located at the Brodmann area centroids. The upper and lower rows indicate significantly higher (red lines) and lower (blue lines) connectivity values in patients (PAT) compared to controls (CON), respectively.

Figure 3. Global network degree (K), clustering coefficient (C) and efficiency (L) as a function of threshold values for each frequency band. The error bars represent the standard error of the mean with 95% confidence intervals and * indicate significant differences between PAT and CON.
Our results should be interpreted with caution because of the low sample size, limited number of EEG segments, and short EEG data lengths. Apart from technical limitations, collecting long EEG recordings from children under age 16 is difficult because they cannot adequately follow instructions. Further studies with larger samples and longer EEG recordings are required to confirm our findings.
Referencres
Adebimpe, A., Aarabi, A., Bourel-Ponchel, E., Mahmoudzadeh, M., Wallois, F., 2015. Functional Brain Dysfunction in Patients with Benign Childhood Epilepsy as Revealed by Graph Theory. PLoS One 10, e0139228.
Adebimpe, A., Aarabi, A., Bourel-Ponchel, E., Mahmoudzadeh, M., Wallois, F., 2016. EEG Resting State Functional Connectivity Analysis in Children with Benign Epilepsy with Centrotemporal Spikes. Front Neurosci 10, 143.