Detection of EEG transients in neonates
Electroencephalography (EEG) is characterized by ongoing background rhythms and transient events with variable amplitude and frequency. EEG transients are brief, non-periodic activities that are distinct from the background activity, and can be classified based on their duration. In adults, EEG transients are often associated with some form of brain dysfunction, and their polarity, amplitude, shape, and spatial distribution can provide insight into the location and severity of the abnormality. For example, epileptiform discharges such as interictal spikes and sharp waves (Fig. 1) are the most common EEG transients in adults, and play a key role in the diagnosis of epilepsy (Smith, 2005).
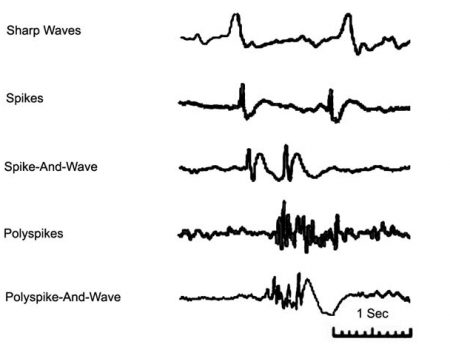
Figure 1. Epileptiform discharges in adult EEG.
In neonates, EEG background activity is composed of both continuous and discontinuous patterns. The discontinuous EEG background activity is characterized by long periods of very low amplitudes, interrupted by high-amplitude bursts of variable frequencies. As preterm and full-term newborns mature, their cerebral activity is marked by a wide range of age-dependent electrophysiological properties. This maturation process is accompanied by changes in the temporal and spatial characteristics of electroencephalographic events. In newborns, the presence or absence of certain EEG physiological events can indicate the temporal involvement of cerebral neural networks. In preterm and full-term infants, there are several easily recognizable physiological EEG transients with short durations (<1 sec), such as delta brush and temporal theta activities (Fig. 2a and b). Pathological EEG transients, on the other hand, are electrographic signs of local disturbances in brain network functions, such as positive rolandic sharp waves (Fig. 2c). These transients provide valuable insight into the development of the infant brain.
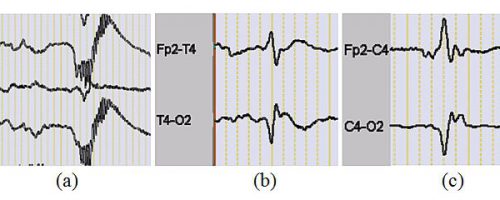
Figure 2. (a) delta brush patterns, (b) premature temporal theta, (c) positive rolandic sharp waves.
The detection of EEG transients in neonates is a complex and time-consuming task due to their morphological variability and the ever-changing temporal and spatial characteristics of EEG background activities. Traditionally, EEG experts have relied on visual inspection to detect EEG transients based on their morphology and spatial distribution. However, this procedure is both labor-intensive and requires highly trained neurophysiologists. In 2009, our research team developed a semi-automatic EEG transient detection system to detect physiological and abnormal transients in neonatal EEG (Fig. 3). This method involves the selection of a template (an EEG transient of interest, Fig. 4) to capture the spatio-temporal characteristics of the events to be detected. This template is then fed into a coarse-to-fine temporal event detection procedure to identify single-channel EEG event candidates. Finally, a coarse-to-fine spatial event selection algorithm is used to select and group EEG event candidates based on their spatial characteristics. The ultimate goal of this system is to accurately identify event groups with similar temporal characteristics and spatial distribution.
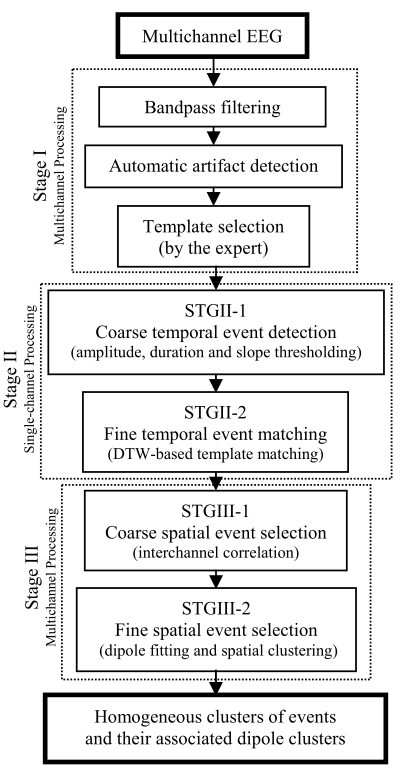
Figure 3. Block diagram of the spatiotemporal EEG event detection system.

Figure 4. Temporal features calculated for each event: half-wave amplitudes (Ahw1, Ahw2), event amplitude (Aw), half-wave durations (T1, T2), half-wave slopes (S1, S2).
In the preprocessing step, multichannel EEG signals are first band-pass filtered between 0.5 and 70 Hz to eliminate low-frequency artifacts and high-frequency noise. Additionally, a notch filter is applied to suppress 50 Hz power-line interference and reduce false positives due to artifacts. To further ensure accuracy, three of the most common artifacts - movement, electrooculogram (EOG), and electromyogram (EMG) - are identified using an artifact detection algorithm. This hierarchical time-domain procedure involves the application of both coarse-tuning (feature thresholding) and fine-tuning subroutines (shape matching). On a single channel basis, peaks and troughs are determined using a detection algorithm based on zero-crossings of the first derivative of the filtered EEG signal on the template channel. All waves exhibiting the same polarity as the template are then selected. Subsequently, the morphological features (Fig. 4) of the preselected waves are determined, providing a set of relevant features with maximum discrimination power for temporal event detection. All events exhibiting similar features to those extracted from the template within specific threshold ranges are selected and saved for further analysis using the fine-tuning procedure. Finally, a dynamic time warping (DTW)-based template matching method (Fig. 5) is used to achieve maximum correlations between the template and selected event candidates, allowing for the selection of transient events exhibiting maximum similarity in shape with the template for spatial event detection.
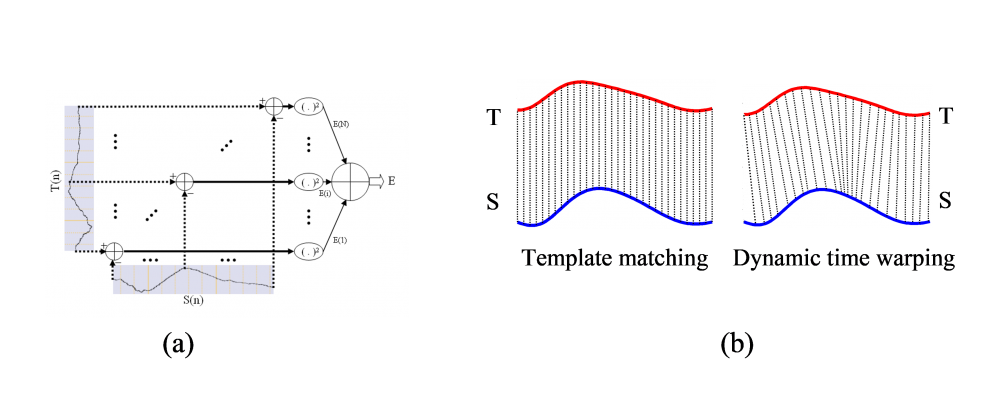
Figure 5. (a) Summed squared error between the template (T) and a segment of signal (S), (b) typical template matching, one-to-one alignment on the time axis versus dynamic time warping non-linear alignment with a warped time axis.
To achieve maximum sensitivity and selectivity, we employed a hierarchical spatial coarse-to-fine selection procedure, which leveraged inter-channel correlations to eliminate single-channel isolated events. This step involved evaluating each candidate selected at the fine temporal event matching phase one by one. Template-like event candidates were kept if the interchannel correlations between the template channel and other channels within each event's time window exceeded a predefined threshold. Finally, a fine spatial event selection procedure was used to identify and classify events based on their spatial features, which were computed using a dipole fitting method (Fig. 6).
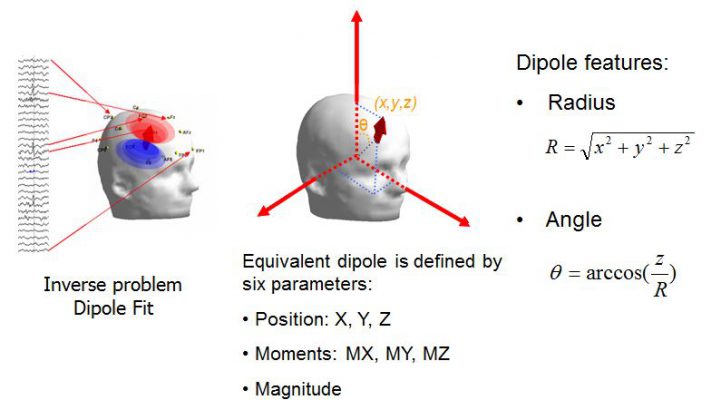
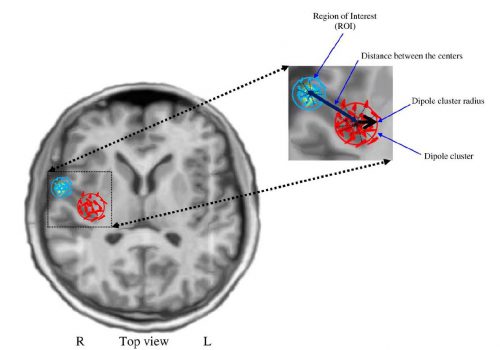
Figure 6. Definition of the parameters used for the spatial clustering. R: right, L: left.
To evaluate the spatiotemporal event detection system, high-density (64 electrodes) EEGs were recorded from four neonates (aged 36–43 weeks) and six older children (aged 1.5–13 years) to identify focal spikes or sharp waves. The system was evaluated at two levels. First, the performance of the temporal and spatial event detection subsystem cascaded with the coarse spatial event selection stage was computed in terms of sensitivity and selectivity. The events detected by the system were then compared with those marked by experts, yielding mean sensitivities of 84.9% and 91.9% and mean selectivities of 86.3% and 90.6% for neonates and older children, respectively. The results of the spatial clustering for Patient I and all patients are illustrated in Figs 7 and 8, respectively. For further information, please refer to Aarabi et al. (2009).
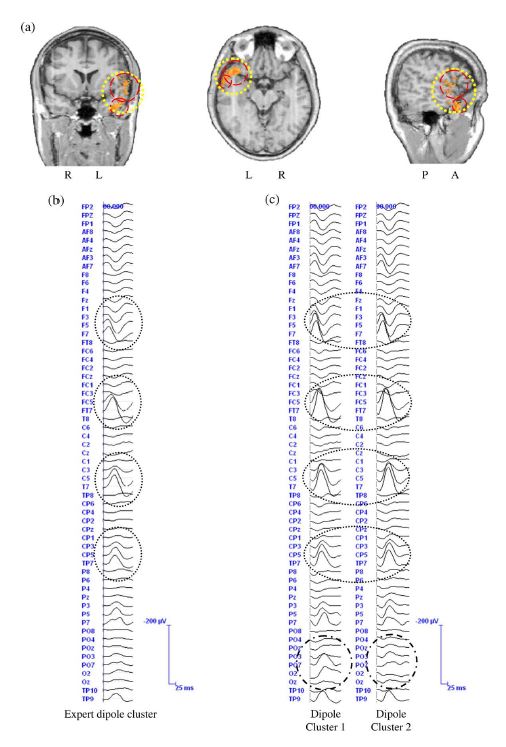
Figure 7. (a) Results of the source reconstruction for the events detected by the experts and by the automatic method for Patient I. The dipole cluster for the expert-marked events is represented by a large dotted circle. The dipole clusters for the system-detected events are represented by dashed circles. The average event for the expert-marked and the systemdetected events are shown in (b) and (c), respectively. The dotted ellipses show the channels, on which the spatial clustering of the dipoles could clearly increase the SNR of the average time series, compared to the expert-detected events. The dot–dashed ellipses show the differences between the average time series resulting from spatial clustering of the dipoles. R: right, L: left, A: anterior, P: posterior.

Figure 8. Spatial clustering of the dipoles reconstructed for the events detected by the system. For each patient, the ROI is represented by a dotted circle and the dipole clusters are shown by dashed circles. R: right, L: left.
References
Aarabi, A., Kazemi, K., Grebe, R., Moghaddam, H.A., Wallois, F., 2009. Detection of EEG transients in neonates and older children using a system based on dynamic time-warping template matching and spatial dipole clustering. Neuroimage 48, 50-62.